Introduction In the realm of SQL Server, managing data involves various intricate processes that impact the integrity and consistency of stored information. Critical aspects like locking, data modification, and committing changes play a pivotal role in ensuring the...
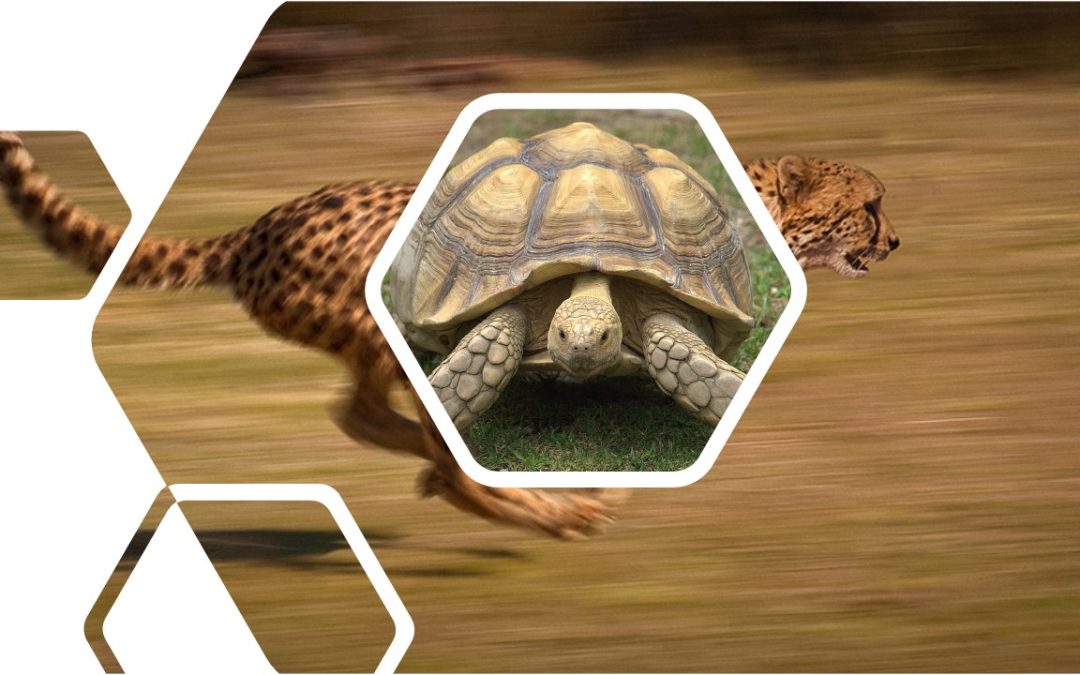
Exploring Locking in SQL Server
Introduction
In the realm of SQL Server, managing data involves various intricate processes that impact the integrity and consistency of stored information. Critical aspects like locking, data modification, and committing changes play a pivotal role in ensuring the reliability and stability of a database system in large data workloads.
Understanding Locking
In SQL Server, locking plays a fundamental role in managing concurrent access to data. Locks control resource contention, ensuring the isolation and integrity of data modifications performed by different transactions. There are different types of locks, such as shared locks, exclusive locks, and update locks. For instance, when a SELECT statement reads data, it acquires shared locks, while UPDATE or DELETE statements acquire exclusive locks to prevent other transactions from modifying data concurrently.
Data Modification
When modifying data in SQL Server, it’s essential to consider the effects on the integrity of the database. Operations include INSERT, UPDATE, and DELETE. For example, an UPDATE statement alters existing records, while DELETE eliminates records from the database. Inserting new records impacts the primary key constraints and relationships within the database.
Committing Data
Committing changes in SQL Server is an essential step in finalising modifications to the database. The COMMIT statement ensures that all changes made by a transaction are successfully applied and persisted in the database. If changes are not committed, they remain in a pending state and are not visible to other transactions. Conversely, the ROLLBACK statement reverses modifications made by a transaction if there’s an error or an intentional rollback.
Practical Examples
Example 1 – Locking Scenarios:
Consider a scenario where two users concurrently access the same table. User A performs an UPDATE operation on a set of records while User B simultaneously attempts to modify the identical records. This scenario might lead to contention due to conflicting locks, impacting data consistency.
Example 2 – Data Modification and Committing:
Assume a banking system where a transaction involves transferring funds between two accounts. The operation requires updating the balance in both accounts while ensuring the atomicity of the transaction. Committing the changes ensures that the funds are transferred accurately and durably stored in the database.
To prevent table locks in SQL scripts or statements, consider the following strategies:
- To improve concurrency and minimise locks on tables, consider using snapshot isolation levels like READ COMMITTED SNAPSHOT or ALLOW_SNAPSHOT_ISOLATION. These levels store a version of the data when changes are made, which allows for concurrent read access. Using snapshot isolation, you can reduce the chances of blocking other users from accessing the same data.
- Implementing table partitioning divides large tables into smaller, more manageable units based on criteria such as date ranges. This strategy helps distribute data across multiple partitions, reducing contention and the likelihood of table-level locks.
- Column store indexes can significantly enhance the performance of queries for large workloads, particularly regarding analytical queries on extensive tables. They are highly efficient when dealing with large-scale data warehouse scenarios where analytical queries aggregate vast amounts of data. By organising data by column rather than row, these indexes reduce I/O and memory requirements, resulting in faster query execution and reducing the impact of locking.
- Another effective strategy is utilising the column store index’s compression and storage capabilities. Compared to traditional indexes, column store indexes have better compression, allowing more data to be stored in memory and reducing the need for frequent I/O operations. This can significantly improve query performance without causing locks due to reduced disk access. Bear in mind that the decision to implement column store indexes in a data workload is context-dependent and varies based on factors such as the size of the dataset, the nature of queries executed, and the performance requirements.
Conclusion
Understanding the intricacies of locking, data modification, and committing data in SQL Server is crucial for maintaining data integrity, concurrency, and overall database performance. Practising these concepts with a comprehensive grasp of their implications ensures efficient and reliable database management.
This article aims to provide a broad understanding of the core concepts surrounding locking, data modification, and committing data in SQL Server, along with practical examples to illustrate their significance in database management.